PROVIDENCE, R.I. [Brown University] — Three Brown University professors are recent recipients of National Science Foundation Early Career Development (CAREER) Awards, the agency’s most prestigious award for junior faculty scientists.
Baylor Fox-Kemper, assistant professor of geological sciences, will receive $594,000 for work aimed at improving global circulation models of Earth’s oceans. Thomas Serre, assistant professor of cognitive, linguistic, and psychological sciences, will receive just over $500,000 for work aimed at understanding brain mechanisms behind the human visual system. Erik Sudderth, assistant professor of computer science, will receive $509,000 to support his work on independent machine learning and large datasets.
Awards for Fox-Kemper and Sudderth are beginning this spring; Serre’s award began in September.
Improving climate models
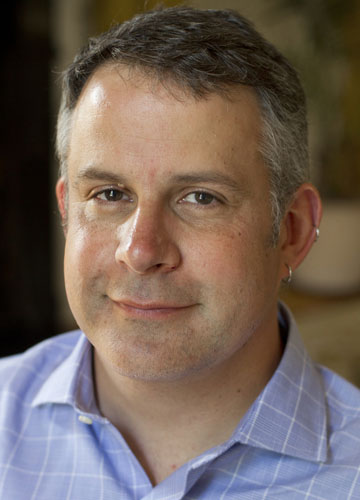
Scientists simulate circulation in the oceans to help understand the role oceans play in regulating climate. Current simulation methods, however, have a hard time incorporating the effects of small-scale currents and eddies into global models. Those phenomena, though occurring on the scale of only tens of kilometers, can have a significant influence on how heat and carbon dioxide are exchanged between the ocean and the atmosphere. Because these simulations are a primary tool in projecting future climate, including human-induced changes, accuracy is of utmost concern.
Fox-Kemper is developing mathematical and physical analyses that improve the representation of small phenomena in global models. Ultimately, he hopes these higher-resolution models will give scientists a better understanding of the oceans and enable better predictions about the pace and consequences of climate change.
The grant also includes a substantial education and outreach component. Fox-Kemper will give a series of public presentations throughout the state in collaboration with Rhode Island Save the Bay. To enrich those presentations, Fox-Kemper will work with Brown’s Science Center to produce a series of short animated films, dubbed SciToons, to help explain the physics of climate. A related sci-toon produced by the Science Center is available online.
“I am looking forward to the outreach activities in my grant because I am new to Rhode Island. I arrived only in December, 2012,” Fox-Kemper said. “I moved from a land-locked state, where interest in the oceans is more abstract. I am eager to meet other people working to ensure ocean sustainability and conservation and to meet students and citizens across Rhode Island who want to know more about climate and oceans.”
Understanding how we see
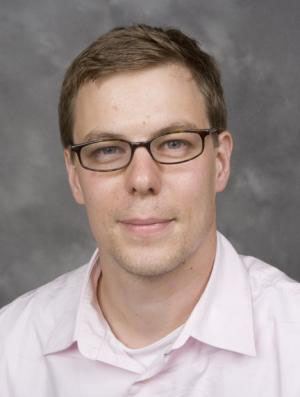
Thomas Serre’s lab develops computational models of the primate visual system. The eventual goal is to build computer algorithms that enable machines to mimic the way humans see.
Serre’s CAREER work focuses on one important piece of the human visual puzzle: The mechanism through which we recognize objects.
Within milliseconds of laying our eyes on a visual scene, our brains begin categorizing objects in the visual field. To understand how that works, Serre uses experiments in which pictures are flashed before people’s eyes for vanishingly brief periods. The pictures flash so quickly, in fact, that people may not be aware they have seen anything, yet they are still able to answer questions about objects in the picture with surprising accuracy.
“It’s interesting because it shows that the visual system is extremely fast and can process visual information under severe time constraints, possibly without cortical feedback or shift of attention,” Serre said.
Serre hopes to use data from these experiments to model what happens in the brain during the first 150 milliseconds of visual processing before more complex visual processes take place. It would be a significant first step toward more complete algorithms that mimic human vision.
Such algorithms could be put to use in self-driving cars, robots that can explore unmapped terrain autonomously, or a myriad of other applications. For instance, Serre has developed a computer vision system for the automated analysis of animal behavior based on the organization of another part of the visual system, which performs on par with human manual scoring.
“Once you have a working model of the visual system,” Serre says, “you can use it to solve all kinds of real-world problems.”
Machine learning and large datasets
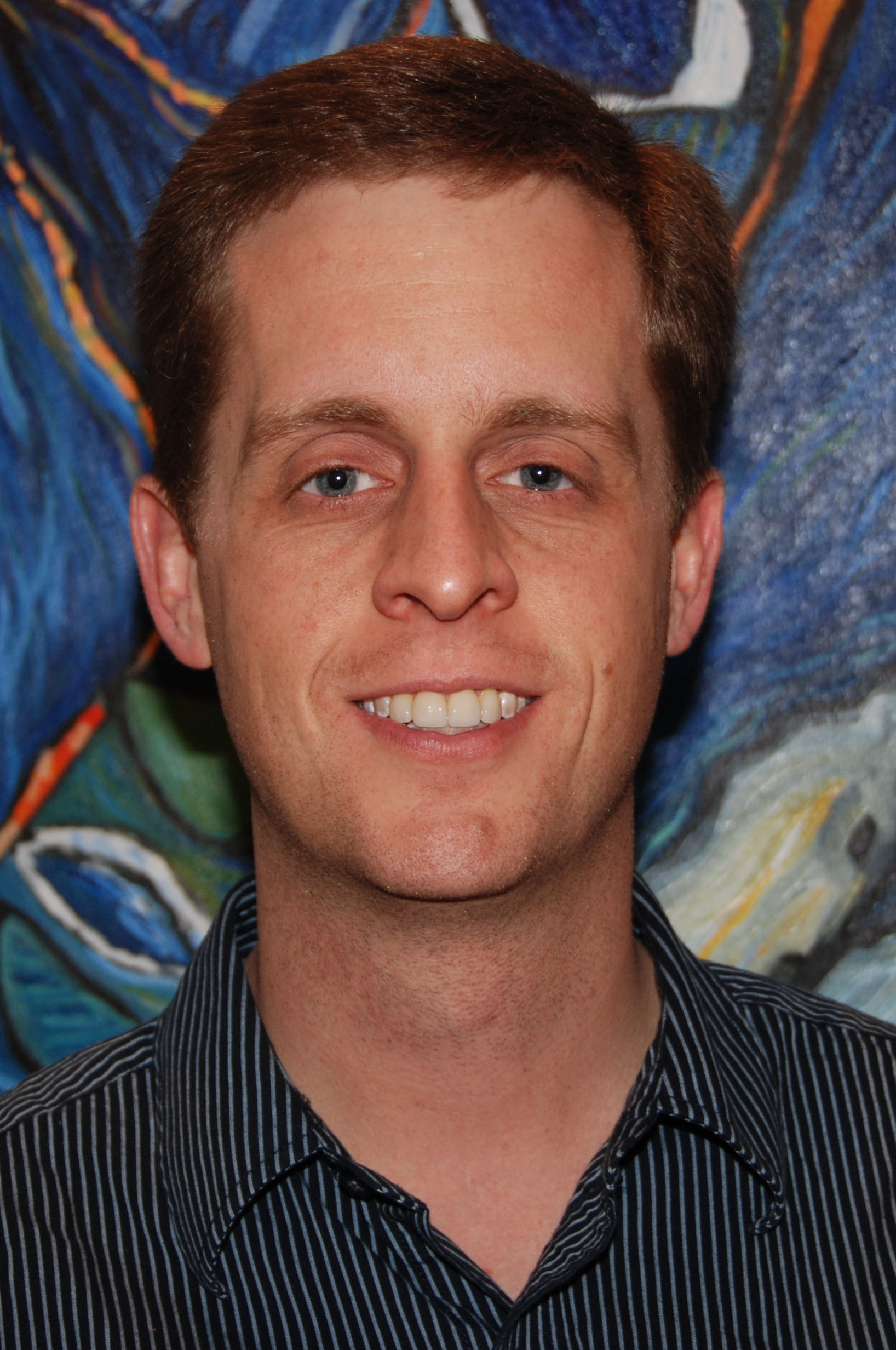
Erik Sudderth’s CAREER grant focuses on news ways of using machine learning to analyze large datasets, which could include anything from the videos available on YouTube to archived issues of the New York Times. Standard machine learning algorithms rely on human users to specify manually all the data representation which will be learned. For example, an algorithm designed to find faces in photos would need to be shown numerous examples of faces, and given a list of suggested cues for discriminating faces from background.
“This puts real limits on what can be learned from even very big datasets,” Sudderth said, “because the model’s structure has to be manually specified by experts.”
Sudderth is working on a modeling approach that allows the structure of the model to be determined by the data itself. This allows for algorithms that are capable of “unsupervised” learning. Because less manual supervision is needed, such methods are much more broadly applicable.
Applications for models of this kind are almost limitless: helping computers analyze photographs to differentiate objects from their surroundings, allowing robots to determine human cognitive states based on facial expressions, or finding communities within social networks by analyzing patterns of collaboration.
The CAREER funding also supports an education and outreach effort that includes an accessible Python software package to allow for easier data analysis, interdisciplinary research projects involving undergraduate students with training in other sciences or the humanities, and two week-long summer seminars to be held at Brown's Institute for Computational and Experimental Research in Mathematics (ICERM).